The State of AI in Insurance
AI is at a pivotal point in the insurance industry, teetering on the edge of revolutionary change. As technologies mature, we can only expect to see more ingenious applications emerge, fundamentally altering the landscape of the insurance business.
By understanding the current state of AI adoption and maturity across various sectors, insurance companies can better position themselves for the inevitable wave of digital transformation.
The global market for artificial intelligence (AI) in insurance is not just growing—it's booming. In 2022, the market was valued at approximately $4.59 billion, but by 2032 it is projected to skyrocket to nearly $80 billion. That represents a Compound Annual Growth Rate (CAGR) of 33.06% between 2023 and 2032.
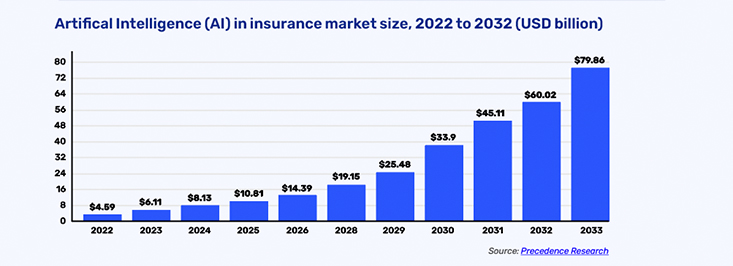
AI technology is becoming increasingly widespread in the insurance industry. A recent survey found that 72% of insurers are using AI in some capacity, and this number is expected to grow in the coming years.
The Landscape of AI Adoption Across Insurance Sectors
A recent survey highlighted that a staggering 72% of insurers are already harnessing AI technologies, a figure anticipated to climb even higher in the years ahead. However, the extent of AI adoption varies depending on the line of insurance and the nature of the business.
The Property and Casualty (P&C) insurance sector is leading the pack in AI adoption, especially within commercial lines. This can largely be attributed to the abundance of data available, which AI can effectively analyze to optimize pricing, fraud detection, and claims processing.
In contrast, the life and health insurance sectors are lagging but catching up. Their slower pace is due to having fewer data sets and the relatively recent introduction of AI in these areas. However, as technology matures, these sectors are expected to increase their adoption rates.
Applications of AI in insurance
Yet, the maturity of AI technology in the insurance industry varies depending on the specific application. Some applications, such as fraud detection, are relatively mature, while others, such as claims processing, are still in the early stages of development.
- Emerging: These are newer applications of AI technology that are still being developed and tested but show promise for future impact.
- Low: These applications have some implementations but are not yet widespread. The technology and methods may still be evolving.
- Medium: These applications have seen wider adoption and are closer to becoming industry standards. They offer clear benefits but may still have room for improvement.
- High: These are mature applications of AI, commonly used across the industry. They offer significant benefits and are considered reliable.
The use of AI in the insurance industry is still in its early stages, but it has the potential to revolutionize the way that insurance is delivered. As AI technology continues to develop, we can expect to see even more innovative and sophisticated applications of this technology in the insurance industry.
Artificial Intelligence Technology & Applications In Insurance
The Impact of AI and ML on the Insurance Value Chain
Licensing & Reinsurance > Compliance & Regulatory Affairs > Product Development > Distribution & Sales > Submissions & Data Collection > Underwriting & Rating > Quoting & Binding > Policy Issuance > Premium Collection >Claim Processing & Settlement > Customer Service & Support > Data Analytics & Business Intelligence
Harnessing AI and ML across the entire insurance value chain—from risk evaluation to customer service—insurers can make data-centric decisions that translate to heightened efficiency and profitability. Through intelligent use of these technologies, insurers are better equipped to manage risks, control costs, and deliver exceptional customer experiences while continuing to offer competitive products. Let's delve into how AI and ML are impacting each segment of the insurance value chain:
Licensing & Reinsurance
- Licensing is the process of obtaining permission from a government agency to sell insurance. The goal of licensing is to protect consumers from fraud and to ensure that insurers are financially sound.
- Reinsurance is the process of transferring some of the risk of an insurance policy to another company. The goal of reinsurance is to reduce the amount of risk that an insurer bears.
AI Impact in Licensing & Reinsurance
In the licensing and reinsurance stage, AI and machine learning technologies empower insurers to more accurately assess their risk profiles. This facilitates better risk sharing between insurers and reinsurers, optimizes capital allocation, and even allows for dynamic reinsurance placement based on real-time risk evaluation.
- Better Risk Sharing: Enhanced data and predictive models allow for equitable terms between insurers and reinsurers, leading to better risk-sharing and potentially lower premiums for consumers.
- More Competitive Reinsurance Pricing: Efficient processes and a deeper understanding of risks contribute to more competitive reinsurance pricing, which may also translate to cost savings for policyholders.
- Reinsurance Optimization: By leveraging AI and ML for data analysis and risk assessment, reinsurers can craft more effective and cost-efficient reinsurance strategies.
- Dynamic Reinsurance Placement: AI analytics enable real-time adjustments in reinsurance coverage, making companies more agile in response to changing risk profiles and market conditions.
- Risk-Based Capital Allocation: Advanced modeling assists in allocating capital based on risk, optimizing capital efficiency and enhancing financial stability.
- Automated Compliance and Reporting: Compliance and regulatory reporting are streamlined, reducing costs and risks associated with human error, while also enabling quicker adaptation to regulatory changes.
- Operational Efficiency: Automated processes reduce the manual workload, freeing up resources for strategic planning and customer engagement.
- Resilience and Agility: AI-powered tools enable companies to be more resilient and agile, easily adapting to new market conditions or regulatory changes.
By incorporating these AI-powered technologies, insurers not only improve their risk management and compliance but also make strides in customer satisfaction, operational efficiency, and overall resilience.
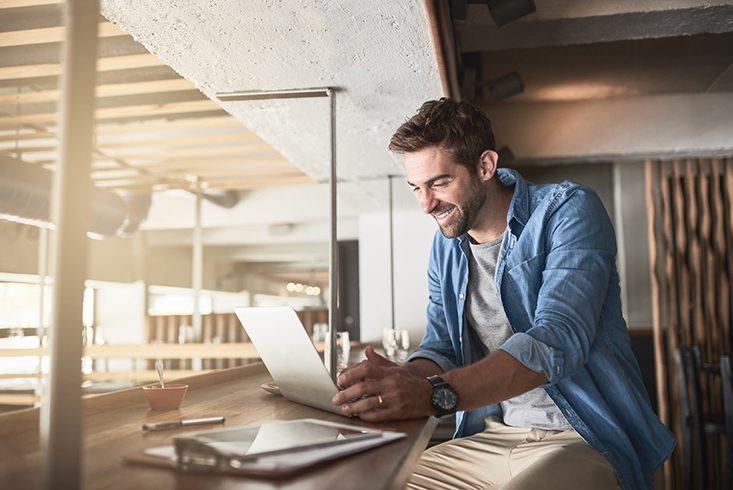
Compliance & Regulatory Affairs
Compliance in the insurance industry is the strict adherence to legal and regulatory guidelines imposed by governing bodies. It's a cornerstone for establishing trust and legitimacy, aimed at protecting both consumers and market stability. Given its complexity, which often involves interpreting intricate legal texts and adhering to ever-changing regulations, compliance can be resource-intensive and fraught with risks of human error or oversight.
Compliance is a complex task that involves interpreting intricate laws and regulations. AI significantly streamlines this process, offering automated regulatory text interpretation, real-time monitoring, and automated reporting, thereby reducing the risk of costly compliance failures. AI-powered compliance tools assist insurers in monitoring and adhering to ever-changing regulatory requirements, ensuring compliance across the value chain.
AI Applications in Compliance
The use of AI in licensing and reinsurance can have a number of positive impacts, including:
- Early Identification of Non-compliant Activities: Anomaly detection algorithms allow insurers to identify and rectify non-compliant activities before they escalate into more serious issues.
- Reduced Risk of Regulatory Fines and Sanctions: Overall, the use of AI for compliance significantly decreases the risk of missing or misinterpreting regulations, which in turn reduces the risk of financial penalties.
- Efficient Compliance Monitoring: With AI’s real-time analytics, compliance monitoring becomes a real-time activity, enabling quick adjustments and ensuring continuous compliance.
- Regulatory Reporting Automation: AI-driven systems automate the generation and submission of regulatory reports, reducing manual errors and saving time for compliance teams.
- Risk-Based Compliance Strategies: AI and ML enable insurers to adopt risk-based compliance strategies, prioritizing resources to focus on areas with higher compliance risks.
- Automated Record Keeping and Audit Trail: AI and ML streamline record keeping, maintaining an accurate audit trail of compliance activities for regulatory audits and reporting.

Product Development
Product Development: This is the process of creating new insurance products. The goal of product development is to create products that meet the needs of customers and that are profitable for the insurer. Product developers conduct market research to identify potential customer needs and then design products that meet those needs. They also work with actuaries to determine the pricing of the products.
The development of new insurance products is undergoing a revolution thanks to AI technologies. From predictive modeling to AI-driven underwriting, the technology allows insurers to create more targeted and financially sound products, often with quicker go-to-market times.
AI Impact on Insurance Product Development
- Faster Iteration: Using simulation models and real-time analytics, companies can quickly refine and iterate product offerings without waiting for long-term customer data.
- Niche Product Creation: Customer segmentation and targeted risk assessment allow for the development of specialized insurance products, catering to niche markets that may have been overlooked.
- Faster Time to Market: Automated compliance checks can significantly speed up the process of launching a new product, avoiding delays that can be detrimental in a competitive market.
- Competitive Differentiation: By using AI to develop more targeted, flexible, and cost-efficient products, insurance companies can differentiate themselves more effectively in a crowded marketplace.
- Data-driven Product Innovation: AI and ML empower insurers to analyze customer data and identify emerging trends, enabling the development of innovative insurance products that cater to specific customer segments.

Distribution and Sales
Policy Distribution: This is the process of making insurance policies available to customers. The goal of policy distribution is to reach as many potential customers as possible. insurers use a variety of channels to distribute policies, including direct sales, independent agents, and brokers
AI significantly impacts how insurance products are distributed. Advanced algorithms can match products to customer profiles more effectively, while chatbots and automated service platforms can handle a range of customer interactions, offering a more personalized and efficient experience.
AI has the potential to revolutionize how insurance products are distributed and sold, both through direct-to-consumer (D2C) channels and via agents. Here's a more detailed look at each:
AI Impact on D2C Distribution in Insurance
- 24/7 availability While Reducing Costs: Chatbots and Virtual Assistants enable insurers to free up call center resources while providing 24/7 service to their customers.
- Personalized Product Offerings: Using machine learning algorithms to analyze customer data, insurers can create highly personalized insurance packages, thereby increasing the likelihood of purchase.
- Reduced cost of customer acquisition: AI can automate many aspects of the sales process, such as initial customer inquiries and lead scoring, thereby reducing the manpower and costs required for customer acquisition.
- Enhanced customer experience: Through personalized interactions, faster query resolutions, and a more streamlined purchase process, AI significantly enhances the overall customer experience.
- Data-Driven Insights: With AI, sales and marketing efforts can be more data-driven, focusing on what actually works rather than what's assumed to work.
- Customer Loyalty: Personalized interactions and quicker query resolutions can improve customer satisfaction, thereby increasing customer loyalty and lifetime value.
- Strategic Pricing: Dynamic pricing engines allow for pricing strategies that can boost sales during low-demand periods and maximize profits during high-demand periods.
- Cross-Selling and Upselling: AI-based recommendation systems analyze customer data to identify opportunities for cross-selling and upselling additional insurance products that align with the customer's needs.
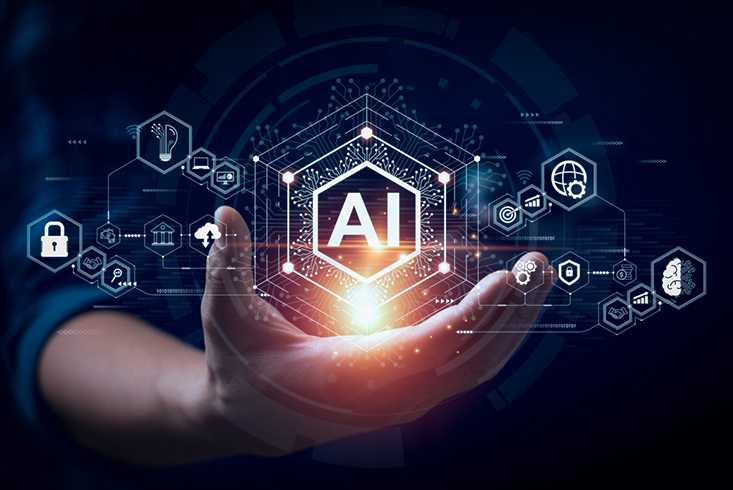
AI in Distribution & Sales for Insurance Agents and Brokers
- Customer Segmentation: Agents can receive AI-driven insights on which customer segments to focus their efforts, improving the ROI of sales initiatives.
- Retention Strategies: By identifying warning signs of client dissatisfaction or departure, AI allows agents to proactively address issues, thereby improving retention rates.
- Qualitative Insights: Natural language generation can convert complex data into easy-to-read reports, providing agents with qualitative insights that are easy to share and act upon.
- Reduced Administrative Burden: Automation of routine tasks frees agents to focus on more value-added activities like relationship-building and strategic selling.
- Optimized Outreach: Machine learning models can predict the best times to reach out to clients or potential clients, thereby optimizing the outreach strategy.
AI technology is revolutionizing the insurance industry's sales and distribution channels, offering distinct advantages for both D2C and agent/broker models.
- For D2C channels, AI enables round-the-clock customer service, personalized product offerings, and a streamlined sales process, all of which contribute to reduced costs and increased sales.
- In the agent/broker model, AI enhances efficiency by automating routine tasks, offers smarter insights through data analytics, and improves customer targeting and personalized interactions.
Whether it's optimizing the consumer experience or empowering agents to be more productive, AI serves as a catalyst for more effective, efficient, and customer-centric practices across the board.
Data Collection: Submissions & Logging
Collecting all the data from the customer and third-parties necessary for underwriting
Submission & Logging: This is the process of collecting and recording information from customers and third-parties. The goal of submission and logging is to create a central repository of information about all policies issued by the insurer. This information can be used to manage the policies, track claims, and comply with regulations.
Collecting accurate and relevant data is fundamental for insurance companies. AI technologies can automate and refine data collection processes, from telematics in auto insurance to wearables in health insurance, providing a more comprehensive understanding of the risks involved.
Submissions and logging of customer data are critical activities that require accuracy, speed, and compliance. Artificial Intelligence (AI) offers a range of tools and technologies that can transform these processes to make them more efficient, secure, and customer-centric.
AI Impact in Submissions & Logging
- Automated Document Logging: Ensure that all documents and data are automatically logged into the appropriate databases or systems, allowing for easy retrieval and maintaining data integrity.
- Streamlined Submission Process: Accelerate the document submission and approval processes, benefiting both agents and customers.
- Reduced Manual Effort and Errors: Minimize the need for manual data entry and reduces the potential for human error, making the process more efficient and reliable.
- Enhanced Data Accuracy: By automating validation and verification, AI increases the accuracy and reliability of the data collected, which is crucial for risk assessments and policy underwriting.
- Faster Decision-Making: Real-time risk assessments allow for quicker and more informed decisions, improving the efficiency of the underwriting process.
- Improved Customer Experience: The use of AI in query handling and personalizing data intake can make the submission process smoother, quicker, and more intuitive, enhancing the overall customer experience.
- Anomaly Detection: Real-time monitoring of transactions to identify anomalies that may signify fraudulent activity.
Underwriting & Rating
Underwriting & Rating is the process of evaluating a risk to determine if the insurance company will insure it and, if yes, then pricing it. Underwriters assess the risk of a particular policy by considering factors such as the applicant's age, health, driving record, and property location. They also use actuarial tables and other statistical data to determine the probability of a claim being made. The price of the policy is then determined based on the level of risk.
The underwriting stage benefits tremendously from AI's predictive analytics and data mining capabilities. Automated algorithms can rapidly and accurately assess risk, leading to more tailored policies and potentially lowering costs for both the insurer and the insured.

AI Impact on Underwriting
- Accuracy: Insurers can more accurately tailor coverage and pricing to each customer's specific needs and circumstances, leading to more accurate and relevant policies.
- Dynamic Underwriting Rules: AI and ML models can continuously learn from new data, enabling insurers to adapt and update underwriting rules in real-time. This ensures that underwriting criteria remain up-to-date and relevant to the changing risk landscape.
- Faster Underwriting Process: By automating data collection and analysis, AI and ML significantly speed up the underwriting process. This enables insurers to provide quicker responses to customers, reducing the time it takes to issue policies.
- Improved Risk Segmentation: ML algorithms identify patterns and correlations within data, allowing insurers to segment customers into more refined risk categories. This fine-grained segmentation enhances the accuracy of underwriting decisions and ensures appropriate pricing for various risk levels.
- Predictive Underwriting: ML algorithms can predict future risks based on historical data and emerging trends. This foresight allows insurers to proactively adjust their underwriting strategies and develop new products to meet evolving customer needs.
- Regulatory Compliance: AI-driven underwriting systems help insurers stay compliant with changing regulations by automatically updating underwriting rules and policy terms to align with legal requirements.

Rating
AI models contribute to a more dynamic and responsive pricing strategy. They enable real-time adjustments based on a multitude of variables, ranging from behavioral data to market trends, leading to more competitive and fair pricing models.
AI Impact on Rating
- Risk Factor Identification: Machine learning algorithms can identify new, non-traditional risk factors that human analysts might overlook, thus refining the premium calculation model.
- Accurate Risk Assessment and Personalized Premium Rates: With the enhanced capability to analyze diverse and real-time data, AI algorithms offer highly accurate risk assessment, leading to personalized premium rates.
- More Accurate Determination of Premium Rates: Predictive and behavioral analytics combined with machine learning offer more nuanced and precise rate calculations.
- Dynamic Pricing Based on Real-Time Data: IoT and telematics allow for real-time data collection that can dynamically adjust pricing and coverage levels.
- Real-Time Premium Adjustments: Dynamic pricing algorithms and IoT devices enable insurers to adjust premiums in real-time, based on ongoing risk assessment.
- Behavior-Based Incentives and Adjustments: Insurers can now offer reduced premiums or other incentives based on good behavior, such as safe driving, thereby encouraging policyholders to minimize risk.
- Location-Based Risk Assessment: Geospatial analytics allow for premiums to be adjusted based on geographic risk factors like flood zones or crime rates.
Quoting & Binding
✓ Quote is sent to the client ✓ Negotiation ✓ When approved, the policy is bound
Once the underwriting is complete and the price set, AI can automate the binding process, ensuring that all the contract terms are met and errors are minimized, leading to a smoother and quicker policy activation process.
Quoting & Binding: Once the underwriter has approved a policy, the next step is to quote a price. The insurer will consider factors such as the policy's coverage, deductible, and term length when determining the price. Once the price is agreed upon, the policy is bound, which means that it is officially in effect.
By leveraging AI and related technologies, insurers can offer a seamless, quick, and personalized experience to both new and existing policyholders. Below is a detailed discussion of the applications and impacts of AI in quoting and binding:
AI Impact on Quoting and Binding
- Reduced Manual Errors and Delays: Automating the quote generation and policy binding processes significantly reduces the chance of manual errors and oversights, which can be costly for both the insurer and the insured. It also minimizes delays, leading to a quicker turnaround time.
- Enhanced Customer Satisfaction: The speed, accuracy, and personalization achievable through AI not only make the process more efficient but also enhance customer satisfaction. Policyholders are more likely to have a positive experience when the process is smooth, quick, and tailored to their needs.
- Scalability: The automation brought by AI allows insurance companies to scale their operations more effectively. They can handle a larger volume of quotes and bindings without a proportionate increase in operational costs.
- Improved Compliance: Smart contracts ensure that all the conditions are met before a policy is bound, helping to enforce regulatory compliance automatically.
- Dynamic Adjustments: Just as AI allows for dynamic pricing in rating, it also permits real-time adjustments during the quoting stage. This means that quotes can change in real-time based on new data or behavior, providing the most up-to-date pricing for potential policyholders.
- Cost Savings: By automating many of the tasks traditionally performed by humans, insurers can significantly reduce operational costs, a portion of which can be passed on to the customer in the form of lower premiums.
Policy Issuance
✓ New business processing ✓ Dec page & Policy package ✓ Send to customer/agent
By employing AI in policy issuance, insurance companies can automate critical functions, like document verification and policy creation, making the process more efficient and error-free.
Policy issuance is the final step in the insurance underwriting process, where the insurance company formally provides the insurance policy to the applicant. This is the point at which the insurance coverage becomes legally binding, subject to the receipt of the initial premium from the insured.
AI Impact on Policy Issuance & Processing
- Automated, Error-Free Policy Issuance: The use of smart contracts and automated document generation ensures that policies are issued quickly and without manual errors, making the process more efficient and reliable.
- Quick Validation of Documents: AI-based document verification can speed up the process of checking the authenticity of required documents, thereby reducing the time between application submission and policy issuance.
- Reduced Manual Intervention: Automation in document verification and policy document generation minimizes the need for manual oversight, thereby reducing the potential for human error and speeding up the issuance process.
- Reduced Operational Costs: Automated systems are generally more cost-efficient in the long run, as they allow insurers to process a larger volume of policies without a corresponding increase in operational costs. This could also lead to more competitive pricing for policyholders.
- Enhanced Customer Experience: The speed, efficiency, and accuracy brought by AI in policy issuance improve the customer experience, making it more likely that customers will complete the purchase of a policy and possibly even become repeat customers.
- Automated Compliance Checks: AI systems can be programmed to ensure that all issued policies are in compliance with current laws and regulations, which is critical for both the insurer and the insured.
Premium Billing
By integrating AI in premium billing, insurance companies can make the entire process more efficient, accurate, and user-friendly. AI can assist in the premium collection process by offering smart reminders, automating payment processes, and even predicting which clients are at risk of defaulting on their payments. Overall, AI offers a transformative approach to the traditionally manual and time-consuming aspects of premium billing in the insurance sector.
Premium collection is the process through which insurance companies collect the agreed-upon fees (premiums) from policyholders to provide them with insurance coverage. Premiums can be collected on various timelines—monthly, quarterly, semi-annually, or annually—depending on the terms of the policy.
AI-powered billing systems efficiently manage premium collections, ensuring timely and accurate payment processing.
AI Impact on Premium Insurance Billing
- Increased Collection Rates: Automated payment reminders and targeted follow-ups can lead to higher collection rates. A more efficient collection process ensures steady cash flow and financial stability for the insurance company.
- Reduced Payment Fraud: Advanced fraud detection algorithms can significantly reduce instances of fraud, such as false claims or identity theft, saving the insurance company potentially large amounts of money.
- Streamlined Billing Process: Automation can make the entire billing cycle more efficient. It minimizes manual intervention, speeds up the process, and reduces the likelihood of errors in billing and collection.
- Enhanced Customer Experience: Automated and timely reminders can enhance customer experience by providing a hassle-free way to manage premium payments. This could lead to higher customer satisfaction and retention rates.
- Operational Efficiency: AI algorithms can process massive amounts of transaction data in real-time, far outpacing human capabilities. This increases the efficiency of the billing department and allows human employees to focus on more complex, value-added activities.
- Compliance and Regulation: AI systems can be programmed to adapt to different billing regulations in various jurisdictions, ensuring that the insurance company remains compliant with local laws regarding billing and collections.
Policy Maintenance & Customer Support
✓ Supporting the customer throughout the policy lifecycle ✓ Call center ✓ Endorsements ✓ Renewals ✓ Cancellations
Leveraging advanced AI technologies allows insurers to provide a more streamlined, efficient, and customer-centric service, covering various aspects like endorsements, renewals, cancellations, and overall customer support.
AI Impact on Policy Maintenance & Customer Support
Customer Communications and Support:
- Deflect to Self-Service:Deflect high volume customer interactions to self-service portals.
- Personalized Customer Interactions: Using AI and ML for tailored communications with policyholders.
- 24/7 Customer Support: Chatbots and virtual assistants for round-the-clock assistance.
- Timely Notifications for Policyholders: Automated alerts for policy details and due dates.
- Quick Claim Status Updates: Real-time tracking of claim status.
- Automated FAQ Assistance: Instant answers to common questions without human intervention.
- Efficient Routing of Inquiries: Directing customer questions to the appropriate department or representative.
- Real-time Assistance with Policy Changes: Immediate support for any policy adjustments.
- Language Support: Customer support in multiple languages to cater to diverse customer bases.
- Proactive Customer Support: Predictive algorithms to anticipate and address customer needs.
- Life-Event Monitoring: Using AI to track significant life events (like marriage or the birth of a child) that might trigger the need for policy updates.
Personalization and Retention:
- Personalized Offers: Tailored endorsement or renewal offers based on customer data.
- Automated Renewal Reminders: Personalized reminders and offers to encourage policy renewal.
- Proactive Identification of Policies Needing Updates: Using predictive analytics to identify and update policies.
- Customer Churn Prediction: Identifying customers at risk of leaving and taking preventive action.
- Increased Retention Rates: Utilizing algorithms to enhance customer loyalty through personalized services.
- Proactive Renewals: Predicting renewal likelihood and offering timely and personalized options.
- Reduced Lapse Rates: Using alerts and analytics to maintain consistent revenue by avoiding policy lapses.
Service Quality and Customer Satisfaction:
- Enhanced Quality of Customer Service: Using sentiment analysis and advanced analytics for continual improvement.
- Language Support: Providing multilingual customer support to cater to a diverse customer base.
Operational Efficiency:
- Efficient Routing of Inquiries: Streamlining customer queries to the right departments or individuals for quicker resolution
By integrating AI into their policy maintenance and customer support frameworks, insurance companies can expect to see significant operational improvements, cost savings, and, most importantly, higher levels of customer satisfaction and loyalty.

Claims Processing & Settlement
The claims process is a formal procedure that a policyholder follows to seek compensation or coverage from their insurance company for a covered loss or event. When an incident occurs that is covered under an insurance policy, the policyholder is entitled to file a claim to recover some or all of the costs associated with that event. The claims process can vary depending on the type of insurance (e.g., health, auto, home, life) and the specific circumstances of the claim.
AI and ML significantly enhance claims processing and settlement by automating tasks, enabling real-time data analysis, detecting fraudulent claims, optimizing settlement amounts, and improving customer engagement. These technologies not only streamline the claims process but also lead to more accurate and efficient settlements, ultimately improving customer experiences and loyalty. Below are some AI applications that are particularly relevant to the insurance claims process:
Data Analytics and Business Intelligence
Finally, the use of AI/ML in data analytics provides insurance companies with valuable insights into customer behavior, market trends, and operational efficiencies. This data-driven approach allows for continuous improvement and innovation across the entire value chain.
Overall, AI and ML technologies optimize operational efficiency, reduce manual errors, and enhance customer experiences throughout the insurance value chain. hese technologies bring automation, efficiency, and data-driven decision-making to various stages of the insurance process, revolutionizing the way insurers operate and interact with customers.
By leveraging these technologies, insurers can deliver more personalized policies, faster claims processing, and improved risk management, positioning themselves for success in the competitive insurance market.
Unleashing the Power of AI and ML: The Critical Need to Digitize Data Collection
In the fast-paced and data-driven world of the 21st century, embracing Artificial Intelligence (AI) and Machine Learning (ML) is not just an option; it's a necessity for businesses seeking to stay competitive and relevant.

However, the true power of AI and ML can only be harnessed when data collection is digitized, leaving behind the limitations of manual or paper-based processes.
You can't run before you can walk - the key to the successful implementation of AI and ML technologies in insurance is to start by digitizing data collection processes. This includes collecting customer information, claims data, policy documents, and other premium-related records in digital-first format.
Once the data is digitized, insurers can leverage AI and ML algorithms with greater accuracy to identify trends, reduce fraud, optimize product and pricing decisions, automate customer service, and provide personalized services.
Embracing digital data collection unleashes the full potential of AI and ML, providing organizations with accurate insights, personalized customer experiences, real-time responsiveness, and the agility needed to succeed in an ever-evolving world. To remain competitive and relevant, businesses must take the decisive step towards digitization and position themselves for a data-driven future.
About EasySend
EasySend is a no-code digital transformation platform designed specifically for insurance and financial enterprises.
Our platform specializes in transforming complex forms into seamless digital experiences. By converting customer data and signature intake processes into interactive digital journeys, we enable organizations to streamline customer interactions, enhance customer experience, ensure data accuracy, and optimize efficiency.
Our solution is trusted by over 100 enterprises, including world-leading carriers, agencies, brokerages, MGAs, MGUs, TPAs, and insurance software integrators. Join the ranks of industry leaders who rely on EasySend to revolutionize their digital transformation processes.